Unlocking the Future of Machine Learning with Data Labeling
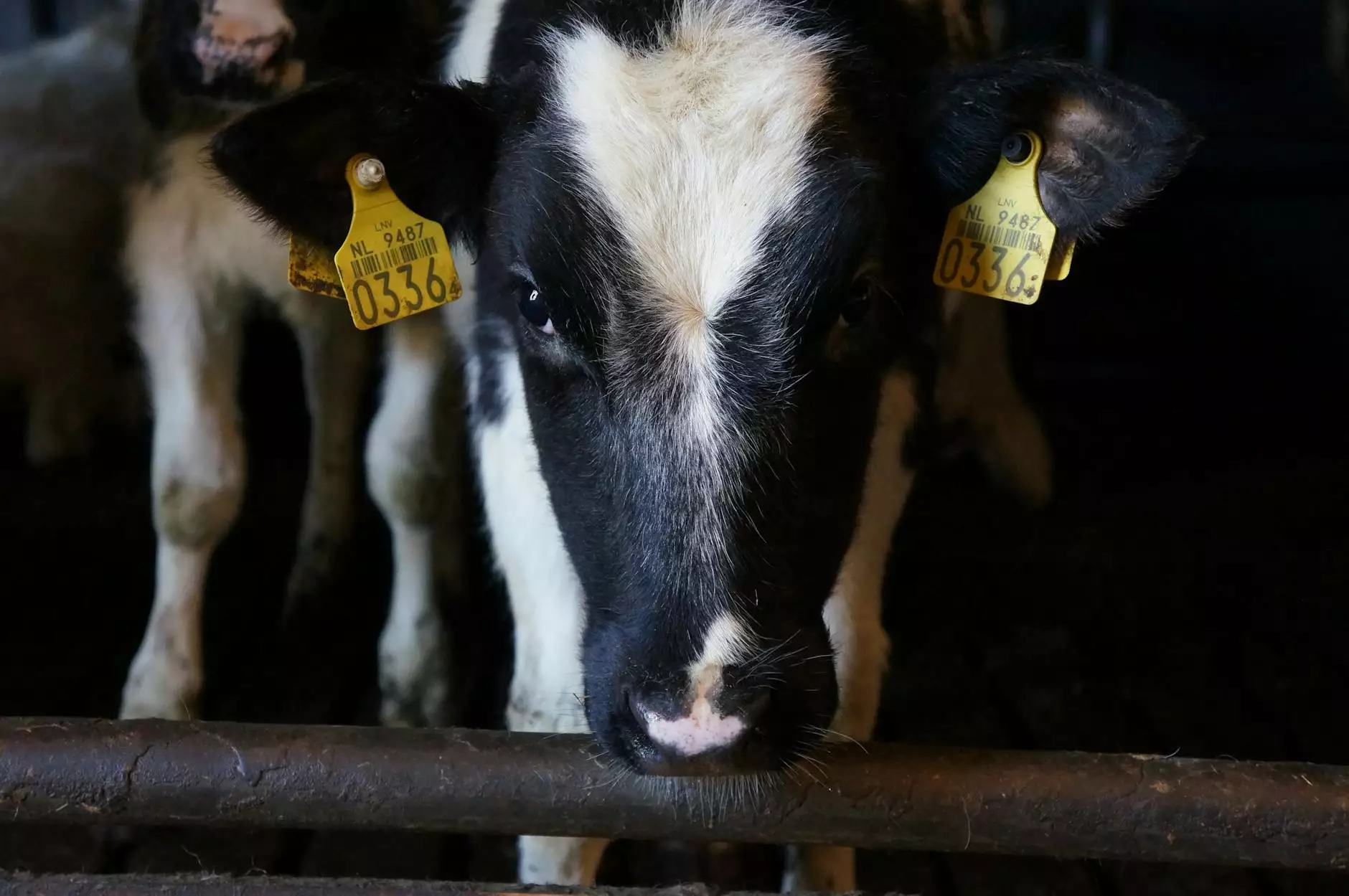
In the world of machine learning, the cornerstone of effective algorithms and models lies in the quality and precision of data. This is where data labeling machine learning comes into play. It is the process of meticulously annotating or tagging data sets to help machines learn and effectively minimize errors during predictive analysis. As businesses increasingly tap into the power of artificial intelligence, understanding and implementing successful data labeling becomes not just advantageous, but essential.
What is Data Labeling?
Data labeling involves the process of associating data with contextual tags that convey its meaning. This is crucial in enabling machines to interpret data correctly. Companies like Keylabs.ai provide sophisticated data annotation tools that simplify and enhance the data labeling process.
- Manual Annotation: Human annotators read, understand, and label data, ensuring contextual accuracy.
- Automated Annotation: Algorithms and AI tools can label data with minimal human intervention, though requiring initial manual training.
- Hybrid Approaches: Combines both manual and automated systems to leverage speed and accuracy.
The Significance of Data Labeling in Machine Learning
Machine learning models are only as good as the data they are trained on. Data labeling significantly impacts model accuracy and overall performance. Here are some key reasons why data labeling is indispensable:
1. Enhances Model Training
The more accurately a dataset is labeled, the better a model can learn patterns and make predictions. Correct labeling reduces ambiguity in the data presented to machine learning models.
2. Increases Accuracy
Having well-labeled data leads to fewer errors during training phases, which directly correlates to higher accuracy when the model is utilized in real-world applications.
3. Supports Various Applications
From natural language processing to image recognition, data labeling is foundational for a broad range of applications:
- Image Classification: Labeling images in datasets to train models for visual recognition.
- Sentiment Analysis: Tagging text data to help models discern emotional tone or opinion.
- Object Detection: Annotating specific objects within images for more complex visual recognition tasks.
4. Facilitates Problem-Solving
Good data labeling can lead to valuable insights and solutions to common industry challenges. Accurate data enables businesses to make decisions based on reliable analytics.
Data Annotation Tools: The Backbone of Data Labeling
To effectively utilize data labeling machine learning, companies need powerful data annotation tools. Here are some critical features that make a tool effective:
1. User-Friendly Interface
An intuitive and accessible interface can significantly enhance productivity, allowing annotators to work more efficiently and make fewer errors.
2. Scalability
As the amount of data grows, a good annotation tool can scale effortlessly to accommodate the increased demand without sacrificing performance.
3. Collaboration Capabilities
Tools that allow simultaneous work on projects by teams enhance collaboration and overall productivity.
4. Quality Control Features
Incorporating quality assurance modules where the accuracy of labels can be regularly checked ensures high-quality outputs.
Choosing the Right Data Annotation Platform
The right choice of a data annotation platform can make a great difference in outcomes. Here are critical considerations for businesses:
1. Specificity to Industry Needs
Different industries have varied requirements. Choose a platform that offers customizable solutions tailored to your specific needs.
2. Cost-Effectiveness
Evaluate the pricing model of the annotation service. A balance between cost and quality is vital to ensure long-term viability.
3. Proven Track Record
Review case studies and testimonials. A platform with a successful history in providing data labeling machine learning services is typically more dependable.
4. Integration with Existing Systems
The platform should seamlessly integrate with your existing data science frameworks to streamline operations.
The Future of Data Labeling in Machine Learning
As technology evolves, so does the landscape of data labeling. Innovations such as active learning and semi-supervised learning are pushing the boundaries of traditional annotation techniques:
1. Active Learning
In active learning, the model queries which specific data points need labeling, thus optimizing the labeling process.
2. Semi-Supervised Learning
This technique combines a small amount of labeled data with a large volume of unlabeled data to improve model learning and reduce operational costs.
3. Automated Solutions
Machine learning techniques themselves are evolving to automate some phases of data labeling, thus increasing throughput and efficiency.
Key Labs’ Offering in Data Annotation
With its robust suite of tools, Keylabs.ai stands ready to assist businesses in achieving their machine learning goals through effective data labeling. Their data annotation platform is designed with cutting-edge technology, customer-centric features, and scalability in mind.
1. Flexible Labeling Solutions
Keylabs.ai offers customizable annotation solutions across various data types, including text, images, video, and audio.
2. Access to Expert Annotators
Users have the option to work with a team of expert annotators who ensure high-quality and precise labeling.
3. Robust Quality Assurance
The platform incorporates multi-layered quality checks that ensure data integrity and accuracy throughout the labeling process.
Conclusion: Embracing the Power of Data Labeling for Machine Learning Success
The importance of data labeling machine learning cannot be overstated as businesses endeavor to harness the full power of artificial intelligence. Companies like Keylabs.ai are paving the way, providing innovative solutions that streamline the data annotation journey and empower organizations to achieve their machine learning aspirations.
As you consider integrating machine learning into your business processes, prioritize the selection and implementation of effective data labeling techniques to ensure your models operate at peak performance. By recognizing the significance of data labeling, you unlock a world of potential that can elevate your business intelligence, enhance decision-making capabilities, and ultimately drive your organization towards success.